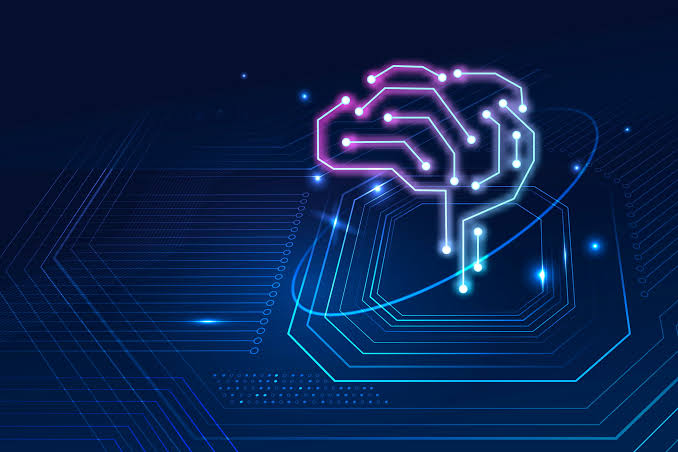
Should professionals have confidence in generative AI when addressing ESG challenges?
The global market of Artificial Intelligence has a double digit CAGR ranging from 19% to as high as 37.7% depending on whose report you read. It is expected to grow at this rate from 2023 to 2032, providing about 133 million jobs and generating more than $15T to the global economy along the way, as over 40% of business leaders have reported increased productivity in AI automation, channeling their creative thinking into more complex tasks and deploying Artificial Intelligence to perform the repetitive simple tasks.
The concept of Artificial Intelligence is one that has been around for decades. In fact, from an etymological standpoint, the conceptual framework of Artificial Intelligence was born in 1950, when a young British Polymath and Computer philosopher Alan Turing published an article titled Computing Machinery and Intelligence. In this paper, Turing asked the question “Can Machines Think?”. He opined that humans use available information and reason to solve problems and make decisions and asked why can’t machines do the same thing? These questions and rationale in reasoning formed the crux for Turing’s paper.
Though he asked the right questions, he was limited to get to work immediately because before 1950, computers lacked a basic component for intelligence which was the fact that they could not store commands but could only execute commands. In other words, computers before 1950 could be told what to do but could not remember what it did and therefore lacked this critical requirement for intelligence. And so, if Turing were to do anything, computers needed to first be changed fundamentally to be able to be considered intelligent.
It was in 1955, that the Proof of Concept for Artificial Intelligence was developed when a Stanford University Professor, John McCarthy organised a conference for top researchers and was funded by the RAND Corporation. McCarthy coined the term “Artificial Intelligence” at this conference and defined it as the “Science and engineering of making intelligent machines”.
Artificial Intelligence according to Howie Baum, a renowned computer Professor, is acomputer system that learns from experience, uses the learning to reason, recognizes images, solves complex problems, understands language and its nuances, and creates perspectives. High-Level Expert Group on Artificial Intelligence (AI HLEG) of the European Commission (EC), defines Artificial Intelligence as systems that display intelligent behaviour by analysing their environment and taking actions, with some degree of autonomy, to achieve specific goals.
Nicole Lakowski and Linda Tucci, both experts in an article on AI defined Artificial intelligence as the simulation of human intelligence processes by machines, especially computer systems. In general, Artificial Intelligence systems work when large amounts of labelled training data are inputted into a computer system, and the system analyses the data for correlations, patterns and using these patterns to make predictions and suggestions. This means that for example, a chatbot that is fed examples of text can learn to generate life like exchanges with people just like the likes of ChatGPT, or an image recognition software can learn to identify and describe objects in images by reviewing millions of examples that had been inputted into it.
Machines will not operate themselves; they need humans to operate them; therefore, it is only those who possess skills in Artificial Intelligence, that will threaten the jobs of those who do not have the skillset for AI.
AI can also be described as machine learning based on helping computers analyse data from a database and learning to predict with more accuracy. So, it is predictive and sometimes repetitive because AI cannot think outside the box, it just makes use of whatever information or data that has been programmed into it, to predict or analyse data.
Let us look at ESG reporting, and the challenges organisations face while reporting their ESG activities, collecting ESG data and measuring their ESG performance. It is a lot of work if this is done manually, as challenges could range from numerous factors.
In fact, one of the challenges organisations and professionals face in ESG reporting ismeasuring and quantifying ESG factors. Organisations drapple with questions like ‘which ESG topics should our company report on? which metrics do we select? And how do we even quantify and measure them. This is because there are no unified or universal standards, and there are lot of complexities and subjectivity that comes to play in measuring and adequately reporting ESG performance.
Another important ESG challenge that bothers professionals and organisations is the issue of ESG data collection. The data collection process is usually complex, and it poses a lot of concerns for professionals because of factors like inefficient and convulated workflows, data complexity and scope and data fragmentation and silos especially having to collect data that are dispersed from one department to the other within the organisation. It is usually a roller-coaster of complexities having to collect data from various departments within the organization especially if there are no standardised uniform methodologies for all departments implemented by the organization.
Another critical challenge professionals face is what to do with the data that has been collected and how to verify them for accuracy, completeness, and reliability. Because of the complex nature of collecting the data, managing, and verifying the data is even more challenging for professionals since the data is being collected from numerous sources. They face the challenge of verifying the data since there is no single source for truth and they may have limited data governance framework.
These challenges and many more are why Generative AI could prove to be beneficial to a lot of professionals as it offers many positive opportunities to bring about efficiencies in ESG Data collection and management workflow. Integrating generative AI into ESG initiatives has the potential to transform various sectors by providing data-driven insights, enhancing risk management practices, driving innovation, and ultimately leading to more sustainable and responsible business practices.
The integration of generative AI and ESG initiatives has the potential to significantly impact various sectors, including finance and supply chain management. Organisations can leverage generative AI and enhance their risk management practices by effectively identifying and mitigating potential risks associated with environmental, social, and governance factors, thereby improving their overall ESG performance. Using generative AI can also drive innovation in the development of sustainable products and services.
But it also raises new questions. To answer these questions, solutions providers need to prioritise the concerns around accuracy, bias, and trust by revealing proactively what they are doing to address them.
As impressive as AI is, it lacks empathy and cannot think outside the box. Let us use the bot Quill for example. This is an AI tool that can write Forbes earning reports. It only contains data and facts that have already been inputted and pre-recorded into the bot. It will only produce the data and facts that it has been programmed with, it is not creative enough to report on something outside its programme, it does not have the tendency to be creative or be empathetic. This is what comes with humanity. AI deals with pre-programmed data and facts, it has nothing to do with empathy. This is why AI tools like ChatGPT and other bots, tend to be monotonous when responding to your queries. Their suggestions and answers tend to be repetitive and streamlined, and this brings us to a very important aspect of Artificial Intelligence which is algorithmic bias.
There will be no Artificial Intelligence without human intelligence. AI does not mean that there is a little human brain that is sentient inside a machine. Humans write the codes that are embedded in AI. Every human has a bias whether it is formed as a result of environment, education, experiences, religion, philosophy, etc; that bias is there. It is what makes us human.
Out of 555 AI models sampled, 83.1% had a high risk of bias.
As long as Artificial Intelligence is a product of human imagination, creativity and innovation, the bias is knowingly or unknowingly deployed into algorithms and codes and this is what drives AI. AI is not free from human bias; this is the reason AI analyses data and events that have been encoded into the system. Even within machine learning, codes are written with human bias every day.
Let us take Nigeria for example where the typical law enforcement agent on the road like the Police has a stereotypical description or definition of who a “Yahoo boy” (Criminals) look like. Policemen seem to think that if you are a young man with dreads, tattoos and piercings dressed in baggy Jeans and loose T-shirts, they brand you a “Yahoo Boy”. Imagine the average policeman in Nigeria writing codes and algorithms aimed at catching criminals, such stereotypes and bias will be reflected in the algorithms and codes the police is developing. And so, when these codes are deployed for AI, it is reflected in the kind of results it produces when it begins to predict crime or analyse criminal data. This means from the very onset, this technology has already been tainted with algorithmic bias.
No robot is free from human bias. Even in deploying AI to detect and analyse crime and catch criminals, the machines are encoded with algorithms and codes that are filled with bias. In an article published by Jeff Hall in March 2023 on neuroimaging-based artificial intelligence models for the diagnosis of psychiatric disorders, he stated that out of 555 AI models sampled, 83.1% had a high risk of bias. In another article published by researchers of the University of Southern California in 2022, they found bias in up to 38.6% of facts used by AI. No AI is bias- free.
A lot of people have said that machines will replace humans, and the world will be ruled by machines. This idea comes from the tenets from Artificial General Intelligence (AGI) that seem to connote that machine intelligence will surpass human intelligence or replace humanity. This AGI concept was borrowed from movies like Star Trek and fancied by Computer Scientists who feel and romanticise about the idea that one day, machines will take over and rule the world. This is a mere statement that cannot happen, as long as machines do not make themselves. If every machine is a product of a human imagination and creativity, no machine will replace any human because machines do not operate themselves.
In fact, AI does not mean that there is a little human brain that is sentient in a machine. No matter how smart AI is taught to be, it can never replace humanity and generate emotions. Dr. Justin Lane of the University of Oxford, a Cognitive and Evolutionary Anthropology Expert who uses AI to help clients predict and map out consumer behaviour, has refuted the theory that machine intelligence will replace human intelligence. In his words, he said “There is something about the continuity of our perceptions within our own minds that cannot be replicated”. “Just because something else has all of your knowledge, it does not make it have your stream of consciousness”.
Just like from the historical antecedents in our industrial revolutions where technology has evolved to now the fourth industrial revolution, what has been transient and constant to changes, has been our skillset. In the 17th century, you needed to be physically powerful to be able to power and operate those steel engines and make iron and build industries. Now you need the skills to be able to operate and control Artificial Intelligence. Machines will not operate themselves; they need humans to operate them; therefore, it is only those who possess skills in Artificial Intelligence, that will threaten the jobs of those who do not have the skillset for AI. So, humans who can use AI will replace humans without AI knowledge. It is still humans taking over the jobs of other humans because they possess a superior knowledge and skills that enable them use AI and gives them that competitive advantage over their peers without AI knowledge. Just as professionals with literacy and numeracy skills replaced illiterates, and those with knowledge of computers replaced those who could not use email in the offices, this is exactly what will happen with those who possess higher skills in the use of AI over their counterparts who do not.
A lot of professionals already feel threatened about their jobs being replaced by AI. Scriptwriters for example are already threatened by the use of AI. However, there is a serious problem of policy regulations. How can plagiarism be detected? There is a need for the development of responsible and ethical use of AI. Hence the need for OpenAI. Meta has developed the Large Language Model AI (LlaMa) that gives researchers the opportunity to use AI for free and fact check each other in how they use the tool.
Scriptwriters for example are already threatened by the use of AI. However, there is a serious problem of policy regulations. How can plagiarism be detected? There is a need for the development of responsible and ethical use of AI.
AI tools like Turnitin, has helped in detecting plagiarism in academic writing by comparing your work with millions of online materials to know the level of originality of your work which is a serious ethical challenge in the use of AI. To what extent is your writing your ‘own’ work? Relying on LlaMa to write essays for us, takes away the originality in us. However, with open access to AI, it gives the opportunity for peers to review your work and detect plagiarism.
There are other ethical issues to be considered too in the use of AI. Let us take self driving cars and the trolley as a case study. What would be programmed into a self driving car? In the event of an imminent danger or an accident, what would it do? Would it kill bystanders to save the occupants or kill the occupants to save bystanders? Who programmes it with what to do andwhat it does? When a human is driving and faces an accident situation, he reacts instinctively. Autonomous vehicles have no instincts, how do they react during accidents? What is the car programmed to do? Are they programmed to hit animals? Or hit people on the sidewalks? Who programmes it to do what? What if they are hacked? What happens?
Let us examine another case study? How would automatic missile systems respond to a false alarm? Would they fire at random? The International Committee of the Red Cross (ICRC) stated that human control must be maintained over weapon systems and the use of force to ensure compliance with international law. What about the issues of Predictability and Reliability in Automatic weapons systems? The ICRC in its report stated that autonomous weapon systems all raise questions about predictability, owing to varying degrees of uncertainty as to exactly when, where, and why a resulting attack will take place. The application of AI and machine learning to targeting functions raises fundamental questions of inherent unpredictability especially as autonomous weapons system self-initiates attacks?
Let’s examine a case study. On March 22, 2003, when the US invaded Iraq, American troops fired a Patriot interceptor missile at what they assumed was an Iraqi anti-radiation missile designed to destroy air defense systems. They acted on the recommendations of their computer-powered weapon system, and fired their weapons in self-defense, thinking they were protecting themselves from an impending missile strike supposedly targeted at them. What their Patriot missile system had identified as an incoming missile was in fact a UK Tornado Fighter jet, and when the Patriot struck the aircraft, it killed two crew members on board instantly. An investigation by the Royal Air Force concluded that the shoot-down was caused by a combination of factors that included: how the patriot missile classified targets, rules for firing the missiles, autonomous operation of patriot missile batteries, and several other technical factors like the tornado not broadcasting its “friend or foe” identifier at the time of the friendly fire. The destruction of Tornado ZG710 was concluded to represent a tragic error enabled by the missile’s computer routines.
Taking the incidence above into consideration, should automatic weapon systems be left to be fully driven by AI or it needs humans to assist in making those hard calls during instances of false alarms, so that another tragic event is avoided? AI should not be focused on replacing humans as it cannot do that, rather, it should be focused on assisting humans to do their jobs more efficiently.
We need to review algorithmic bias when writing codes and programmes for AI tools. It is very difficult to completely eliminate bias in programming, however, we should endeavour to ensure that such bias is kept at a minimal level. We should write computer programmes with empathy and respect the importance of the human life. In fact, Ethics should be included as a compulsory subject in the curriculum for AI, and AI should not be left to be driven only by private capital that may sacrifice ethical concerns at the altar of profits. We should support open access to AI and encourage the ethical and responsible use of AI in research and other professional needs.
Even in Medicine, AI must be deployed with caution to support its use by humans. AI should not be left to run itself 100% without supervision especially in healthcare as it is possible for AI to recommend abortion or euthanasia when humans may make different decisions that would be more humane.
All professionals should be aware of every ethical consideration in the use of AI especially for reporting ESG activities. They should raise concerns, ask questions, and seek for collaborative ways to help the responsible and ethical use of AI in the discharge of their professional duties.
Accuracy, Truth, and Reliability are very key ethical issues that must be considered especially in reporting ESG activities. AI is not here to replace humans, but to help mitigate the challenges humans face in their day to day professional and personal lives. More funding should be budgeted into supporting the development of Ethical research works in the use of AI.
_____________________________________
Wole Abu, Chief Executive Officer of Liquid Intelligent Technologies Nigeria is a Sixteenth Council Senior Fellow